5 Challenges of Building Analytics for Your App from Scratch
Consider these five common challenges as you evaluate whether to build, buy, or partner—or a blend of these options—to deliver analytics to your customers. Read to learn more.
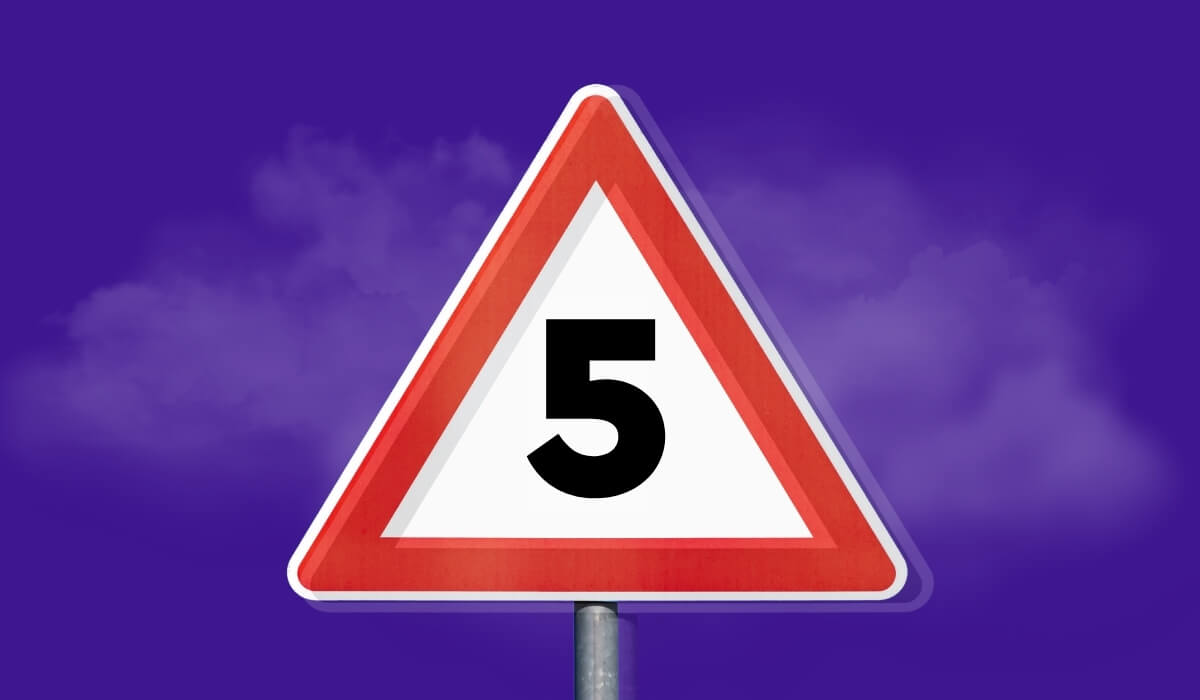
Insightful, user-friendly analytics—customers want them, and product leaders are under increasing pressure to provide them. Even once you’ve decided to move ahead with a project to deliver analytics, a crucial question remains: Will you build, buy, partner, or blend?
People tech vendors know that building from scratch offers complete control and endless customization, but it comes at a steep cost in terms of time, expertise, and resources. And you’ll be on your own to overcome the key obstacles that stand between you and getting analytics into the hands of your customers.
You may be exploring generic BI platforms that help you get to market faster. This comes with its own problems—you’ll need to customize it to your people-specific use cases. All while your team learns to optimize, test, deploy, and maintain across your new suite of tools you need to ingest, process, store, enrich, and serve.
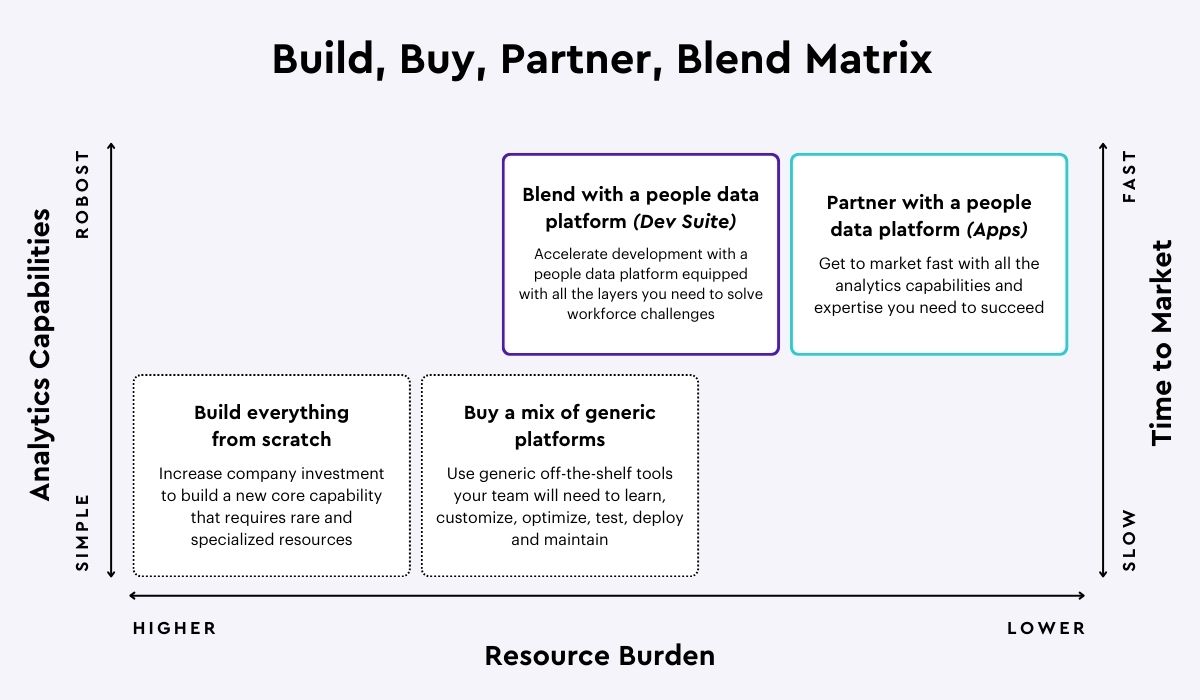
Use this matrix to assess whether to build, buy, partner, or blend to deliver analytics in your app.
5 common people data challenges
Undertaking the task of building your own analytics solution is more complex than you can anticipate. As you evaluate what combination of building, buying, partnering, or blending is right for you, consider these common challenges that arise when launching people analytics.
1. Building an analytic model around people
People data is unlike any other data. Certain employee attributes are fixed and remain constant over time. For example, things like employee ID, birth date, and hire date generally don’t change. However, a lot happens over the course of the employee lifecycle. Events like role changes, reorganizations, and pay changes take place at various point in time before, during, and after their tenure at an organization. No single relational database could account for all of these changes and the myriad ways one employee’s journey differs from another’s. And to accurately perform analysis, you need a historical trail of everything that has happened.
This is where the design of your analytic model is exceptionally important. A robust people analytics solution takes into account all of the things that can happen to an employee over time, independent of the core attributes of that employee, and has baked-in calculations that accurately measure these changes over time.
Key questions to consider:
Do we have the resources and expertise to build this kind of analytic model?
Is analytics part of our core competencies, or will we need to hire a new department to manage this?
2. Handling changes on the fly
Innovation never stops. As your application evolves to exceed customer expectations, you’ll want to make updates based on customer feedback, add new functionality, and evolve and deepen the user experience.
This requires the ability to prototype and test without downtime and end-user impact. And if something does goes wrong, you need to be able to roll back changes to a previous version. It’s critical that these changes are made across all the components of your data platform architecture or your customer may find errors in the front-end. Though tedious, if you have a platform that isn’t built for seamless configuration, your teams will have more preparations to tackle with each change.
Key questions to consider:
How frequently will we update our offering?
What resources will we need to ensure smooth updates that don’t interrupt the user experience?
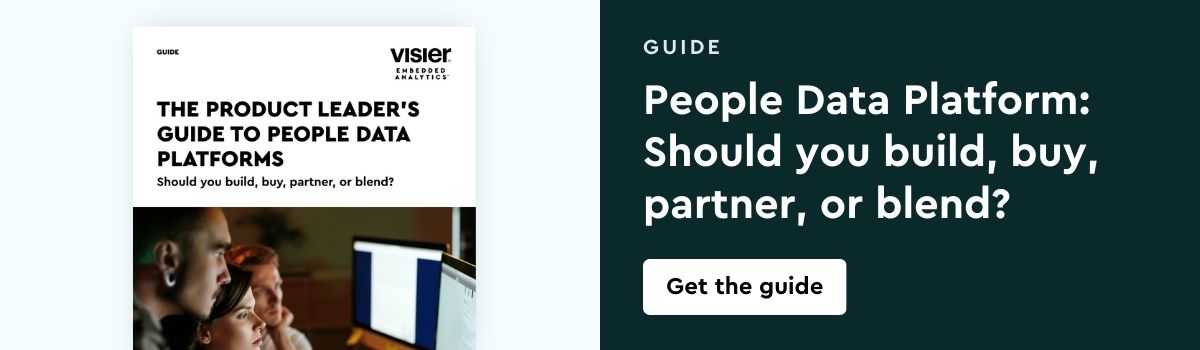
3. Securely distributing analytics at scale
Transitioning to an analytics system introduces great complexity when it comes to access and permissions. Your customers likely will want varying levels of security that they can change and update as users move through the organization, which adds another layer of complexity.
When it comes to distributing people analytics to your users, there are three options. First, you can provide aggregated, anonymized data to everyone. This option provides limited use to your users and comes with security risks. Second, you can provide select groups, like top executives, with sensitive analytic data. This option works well for senior management but fails to provide people managers with critical insights. Finally, you can make tailored analytic data available to users based on identifying attributes like their role, team, or location. This option is the hardest, but most valuable way to distribute analytics to your customers’ workforce.
People analytics is most useful—and most sticky—when it’s available for everyone to use throughout the organization. This requires robust, scalable security model that makes tailored analytic data available to people based on their role, team, location, or other identifying attributes.
Key questions to consider:
How long will it take to set up a scalable, flexible security model?
Can we provide varying levels of security as users move through an organization?
4. Incorporating safe and reliable generative AI
Interest in generative AI shows no sign of cooling down, and customer demand will only increase as more analytics use cases are identified. Incorporating genAI into your analytics will boost uptake by letting even the most inexperienced users ask plain language questions and receive answers they understand and can take action on—no matter their level of data literacy.
But getting started requires training the large language model (LLM) on relevant, well-structured, and anonymized data and managing access to ensure users only receive answers containing the data and insights they have permission to see.
Key questions to consider:
What resources can we devote to generative AI without sacrificing our core competency?
How will we gather the data to train an LLM?
5. Complying with privacy legislation
Working with people data requires complying with existing privacy legislation like the European Union’s General Data Protection Regulation (GDPR) and the California Consumer Protection Act. You also need to be ready to make changes as new legislation is passed. You’ll need to know where data is stored and have a plan in place to minimize data duplication, as well as to delete or anonymize data upon request.
Having a well-defined and documented analytic model with minimal duplication of sensitive data will not only make it easier to comply with increasingly stringent privacy regulations but also reduce efforts to handle any data privacy-related requests.
Key questions to consider:
What do existing privacy laws require of us?
How will we manage data deletion and anonymization?
Get to market quicker with a people data platform
Deciding whether to build, buy, partner, or blend to deliver analytics requires a deep understanding of its alignment with your core competency, implementation time, upfront investment, and total cost of ownership. A people data platform that’s purpose-built for HR and people analytics offers a streamlined solution to accelerate development and reduce costs with more opportunities to incorporate the latest innovations—even with limited resources.
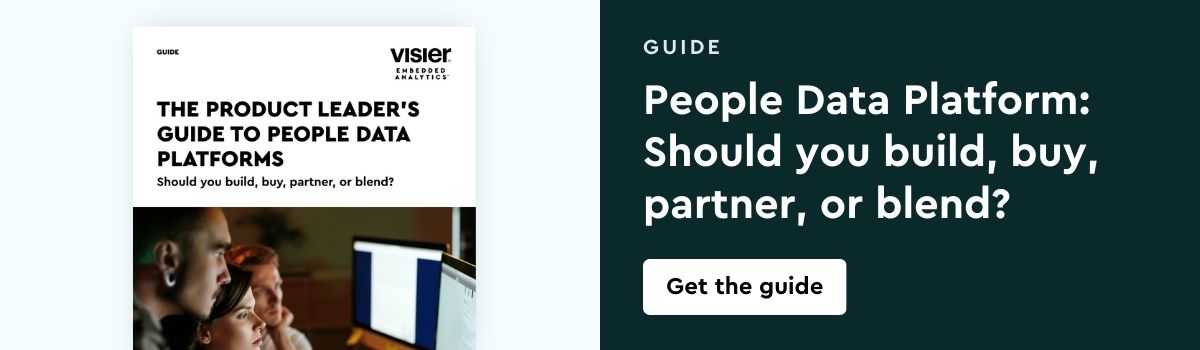