Totally Rewarding Chats | Ep. 27: Workforce Analytics and Total Rewards
Sean Luitjens and Dave Weisbeck discuss how leaders can harness people analytics to drive smarter decisions and shape the future of work.
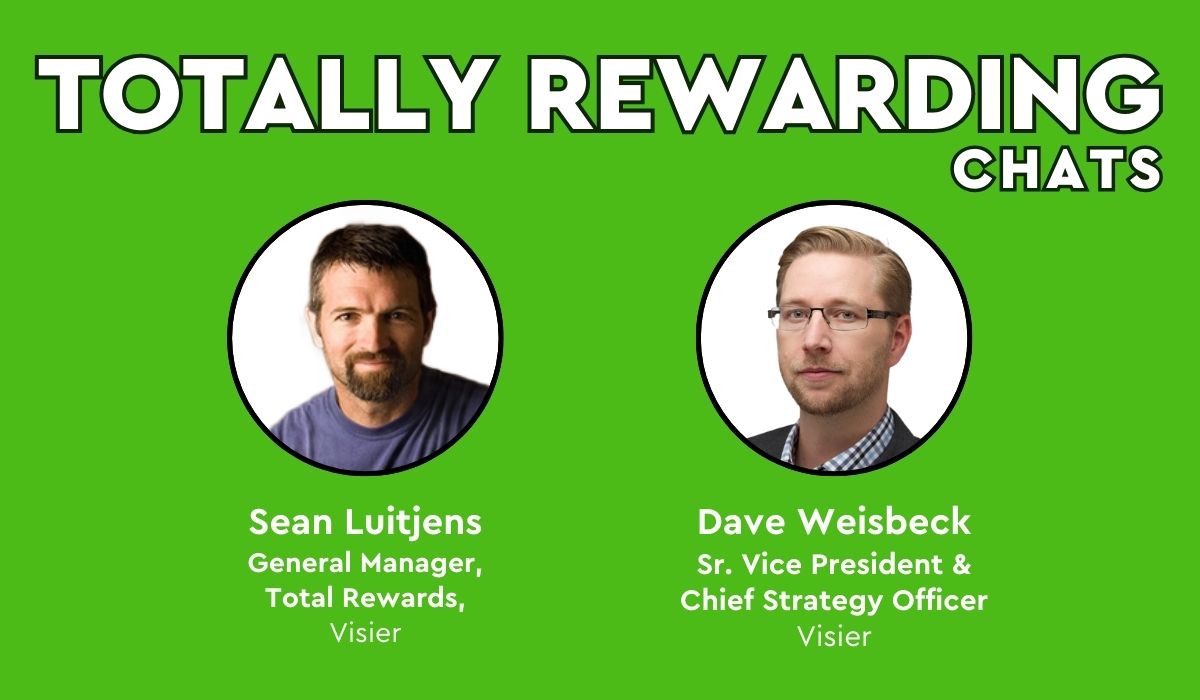
Unlocking the power of people data with Dave Weisbeck
Dave Weisbeck, Chief Strategy Officer at Visier, discusses the transformative role of people analytics in today’s organizations with Sean. From decoding workforce trends to enabling data-driven decision-making, Dave explains how HR leaders and business executives can use analytics to shape strategy proactively. With real-world insights and a future-focused mindset, this conversation explores the intersection of technology, talent, and transformation.
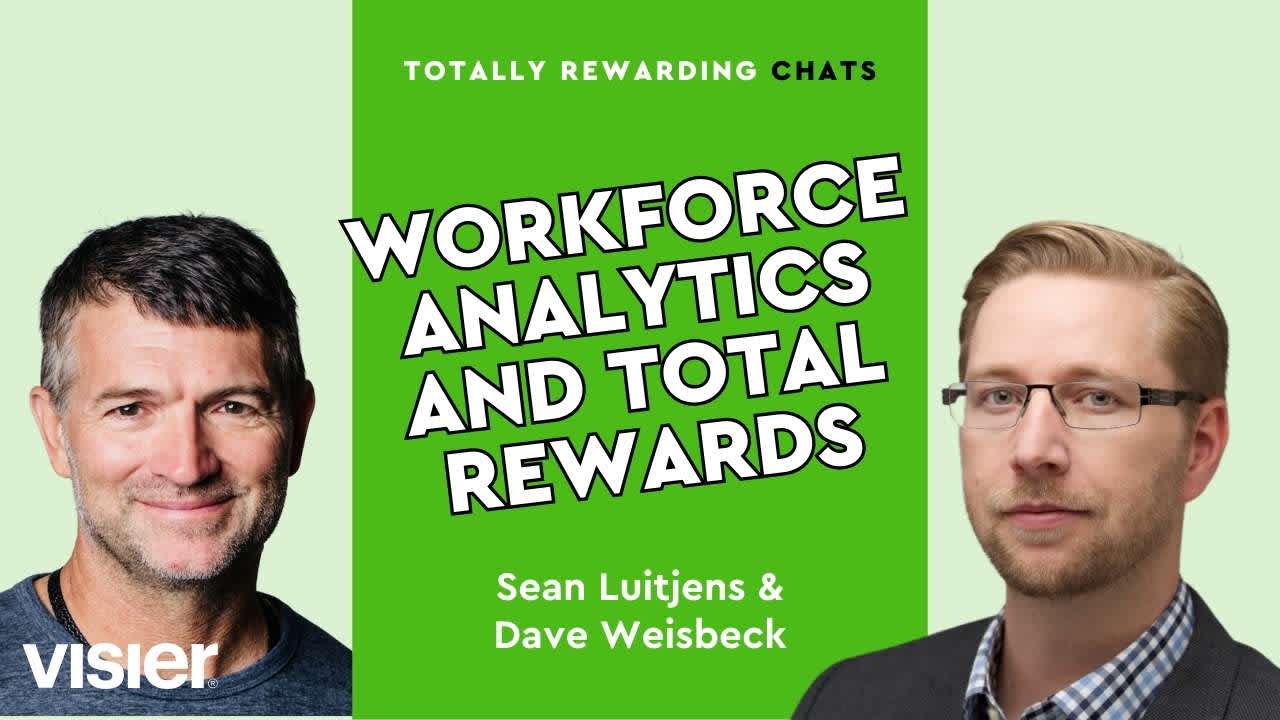
In this episode:
Host, Sean Luitjens, General Manager of Compensation Benchmarks, Visier
Guest, Dave Weisbeck, Sr. Vice President & Chief Strategy Officer, Visier
Episode transcript:
Sean Luitjens
All right, another totally rewarding chat. Getting started, this is going to be a first. We have Dave Weisbeck, who is going to be the first person from Visier to join the totally rewarding chat. So we're only going to be like 20 some odd episodes in and someone finally believe that we might do more than three episodes. So I appreciate you coming on.
Dave Weisbeck
You're from Visier though, so you don't count? But I guess you said joining, so that's fair.
Sean Luitjens
I've been married a long time, so I've heard I don't count a lot, so I don't really count myself. That's just kind of the way it goes. So the first thing is just, so I don't screw up your bio. Give us what you're doing now and kind of how you got there.
Dave Weisbeck
you
Yeah, okay. Fair enough. I can relate. I can relate.
Yeah, so a bit of an intro, who am I and why should anybody care what I say? Well, I'm not certain I can answer the second part of that, but I.
tell you who I am. So Dave Weisbach, Chief Strategy Officer here at Visier. I often joke that's a bit of a title that doesn't mean much to people, but maybe the more important thing is I go back to the beginning of Visier. So for almost 14 years now, I've been trying to figure out how to help people to make smarter decisions about people by bringing more data to those decisions.
But it also pairs for me what really my whole career has been about. So if you go back to the beginning, I've always been a technologist, loved technology, loved the idea of being more analytical, using data, how do you make smarter decisions? How do you bring facts and truth instead of just opinions and I thinks to things that you're trying to figure out. And so I've been doing analytics now for almost 30 years, going way back to products like Crystal Reports, I actually ran that development team.
business objects and looked after the product management there. And then SAP after that. now Visier, I decided I really wanted to do is help people to make the most difficult types of decisions better, which is decisions about people. People are hard to translate into data. A little bit harder than widgets in a warehouse. That's a little easier.
Sean Luitjens (02:15.528)
Yeah, I think the quote I've been using is, humans are highly statistically predictable and amazingly individually unique. And I think trying to figure out those smaller buckets to individuals and make decisions around them is where, you know, we'll talk a little bit about the secret sauce, but, know, instead of just put them on a giant bell curve. So the human capture part is, do you have a hobby or something you do for fun outside of work?
Dave Weisbeck (02:42.682)
I tend to gravitate towards things that are like this blend of art and science, which is maybe why I do analytics, because if you can really tell the story of data well, it matters, not just do the analysis really well. So my hobbies tend to follow that pattern. So two that come to mind, photography is one. There's a lot of like science to, know, balancing shutter speeds. I do something called long exposures, which nobody knows what that is, but I...
I take photos where you open the shutter for eight minutes, which can have some really cool effects. And the other one is cooking, actually, which is the similar type of idea that you really need to understand how it all works and the Maillard reaction, which is how you caramelize things. So there's a science piece, but then there's an art piece because ultimately you want it to look good on the plate and taste good in your mouth.
Sean Luitjens (03:38.172)
That's interesting. So you solved it by doing all that work. I just married an Italian and checked the box. That's how I solved that problem in a totally different way. Yeah. Yeah, I cheated. Yeah, I won't lie. But that's a whole nother episode of how far I married up and or how far she married down. That's definitely one of them. The speed round. All right. You ready? Are you coffee or tea?
Dave Weisbeck (03:42.628)
We'll take that.
Yeah, you took the seating path. I got it, got it.
Dave Weisbeck (03:56.378)
Yeah, it's a whole season more than an episode, you're sure. Okay.
coffee.
Sean Luitjens (04:07.857)
number one, the what?
Dave Weisbeck (04:08.804)
Espresso to be clear actually, you know, I'm gonna break I'm gonna break your speed around espresso to be clear
Sean Luitjens (04:14.49)
Okay, okay, that's fair. We've had a couple people go exactly what type of coffee, which just proves you're a coffee drinker. Number one, if you had to, analytic for companies to look at.
Dave Weisbeck (04:27.29)
geez.
Sean Luitjens (04:32.434)
That's tough for you because you want to weigh them all in your head. I can smell the smoke from out here.
Dave Weisbeck (04:37.118)
Yeah, mean, ultimately it boils down to there are some meta metrics that I'm trying to think of, like what is my favorite one? One of them is, and unfortunately they often are in the financial realm when they come to get to these meta metrics that try to capture the idea of is the business functioning really well?
So you get things like, what is your total cost to serve your customers? Which has a people element, because people do a tremendous amount of that work, but it might have a technology element. Does it work so well in certain industries, know, resources?
But those metrics tend to be the ones I would gravitate towards. They can tell, one metric can tell 10 stories. Those tend to be my favorite, so.
Sean Luitjens (05:30.62)
I should have gone with more, you know, favorite exposure length or something, but you prefer a posh weekend away or like a in the woods, true camping weekend.
Dave Weisbeck (05:44.034)
I am a Canadian born and raised in what's known as the Pacific Northwest and camping is a way of life here. I've never been camping. I love the outdoors. Yeah, give me a cabin.
Sean Luitjens (05:58.822)
It's a whole nother topic we're gonna have to talk about, so, okay.
Dave Weisbeck (06:04.63)
So give me some place that I can have like a lovely fireplace. I'm much happier. So it doesn't need to be posh at all. Like I don't need that. I actually don't like that too much, oddly I find that sometimes too stressful. So I like it simple, but I don't want it to tend.
Sean Luitjens (06:22.824)
Okay, okay, I like that you own it. iPhone or Android? Okay. And the most important question we ask every time, crunchy or creamy peanut butter?
Dave Weisbeck (06:26.904)
iPhone.
Dave Weisbeck (06:34.56)
crunchy. A whole life I've been creamy, but I realized that I my, I don't have much of a sweet tooth. So I can say no to candy any day of the week, like no problem. But chocolate and nuts, when you put those two together, somehow it's just like magic. And I realized almost all of the chocolate and nut things I liked, it's never smooth. It's always crunchy, right? It's full nut or.
Sean Luitjens (07:03.912)
peanut butter cup.
Dave Weisbeck (07:03.93)
it's like. Peanut butter cup might be the one exception to be fair. And so I've like, maybe I should try crunchy peanut butter. And so as I did, I went, I like that better. So I'm a recent
Sean Luitjens (07:15.816)
Okay. Well, there's also Nutella, which is, you know, also a super health food, right?
Dave Weisbeck (07:20.282)
Yeah, but you take Nutella and then you cover it in like ground up peanuts or something like that, or hazelnuts. it's like, that's a fra rocher if you do the hazelnuts.
Sean Luitjens (07:28.616)
Okay. That was the, just so you know, you're the winner so far of the slowest speed round. yeah. Yeah, yeah. So let's start it in. Huh? I don't know. We'll have to figure out if there's a prize. It might be something for you. So define people analytics, which I know is a big thing. So actually in total rewards, the one thing I've learned over the past year or so.
out in the market is if I mention the word people analytics, either, you know, they just think of this huge bucket of things. And so I think it might be helpful if you give a quick overview of how you would define people analytics.
Dave Weisbeck (08:09.208)
Yeah, if I start with kind of like purpose and goal, I would define people analytics as the pursuit of improving how your business operates, functions, whatever you hold out as the measures of success for your business through the smarter decisions you make about people.
And so that would be kind of the purpose statement. What is the pursuit of it in terms of what you actually do? So getting into the weeds of like, okay, yeah, but what's a day in the life of somebody who's doing this? I think you're trying to arm the people who make the decisions about people with the right information they need at the right moment and right time to impact everything across the entire life cycle that employees have.
So from how do we hire better, way up to the front, to how do we develop people in the middle and ultimately retain the ones that are making the biggest difference to our organization. So it spans the entire life cycle. I think sometimes it bumps into things that people might think of as...
in the realm of people analytics, which can be assessments and engagement and some of these softer things. To me, my ultimate definition includes all of those so that we have a comprehensive view of all the intelligence or insight or data or knowledge that we would want to bring to bear to make those smarter decisions about people. Ultimately, that should all be brought together so that we have a unified view.
the information that we have to say how do all of these programs and decisions we make, how do they actually impact our business to be successful through our people.
Sean Luitjens (09:50.152)
And so where does all the data come from? Because all the things you just mentioned, I could probably start to add up and end up with a huge amount of data. So how do companies usually start? And I guess we'll get into best practices or however you want to frame it. So if you're a company thinking, OK, I've got people analytics.
It's a little overwhelming to think about everything from TA to off-boarding to analysis of what happened and why they left and where they went and pulling in all that data over time. Like, one, how do they get started?
Dave Weisbeck (10:21.242)
Yep.
So what I find kind of fascinating about the people realm, I think people in general would say that employees, I'll make it simpler with my language there, that it's not that rich a set of data. And then everybody who goes and looks at it goes, my goodness, like it is super rich because you've got all the core HR systems you would think about your.
core system, your HRIS or HRMS, and you've got then the talent management and the recruiting sides that kind of pair off it. But when you really look at it in big picture, you realize a lot of the systems that are the business operation systems also hold data on people, because it's people who will service things and sell things. so all of that is actually people data. So it's this really expansive set. So then it boils down to your actual question, which is, OK, so then where do you start?
We advocate a methodology called the path without going too far into the weeds on that. The idea is that you really want to start with something that gets the core of the people in your organization, all the employees and the changes in them. One of the things that makes people data really tricky is people change at a much higher rate than say customer data. And so, cause people have careers and aspirations and they, you know,
They go on leaves and they change jobs and they do tons of things. And so it is constantly in flux. So you really want to get a handle on the core of who you all have and what roles and departments and all the rest they're in and how's that changing. And from there, then we advocate you follow a path attached to a more top-down approach, which is, and one of the issues that we're facing as an organization, we're going to pull off our business strategy.
Dave Weisbeck (12:17.826)
and how do people impact that business strategy and go after those. We're growing and expanding, so we need to understand hiring. We need to improve our margins as a business because that's the strategic imperative. Okay, how do we go after a better understanding of costs and where they're coming from? And so you can take different paths based on what the business imperatives are.
Sean Luitjens (12:43.304)
And how long does it usually take? So I mean, it sounds a little blue skies, rainbows and unicorns, you know, when you're like, I'm going to magically pull in this data. And by the way, for those people who were just thinking about the people systems, you just basically said, screw that. We're also going to add all the data from the business that comes in, you know, so how long does it take or is there a best practice to crawl, walk, run into people analytics?
Dave Weisbeck (12:51.652)
Yeah. Yeah.
Yeah, a couple of things for people to think about on this in terms of how long it takes. First, I reject the basis of your question. I'll start there. Analytics is you set up an application. So I'm to put in place an applicant tracking system. There's a project that has a start and an end. And now you have your process in place to how you hiring. Analytics never is that way because every time you, there's always more data.
There's always change in the data you have. Every time you answer a question, and it always leads to two more to have deeper understanding. So always, the first thing I always coach is never think about analytics as a destination. It is always a journey. So you're constantly evolving. And so there's almost like this cultural thing. You got to get your head around of like, this is something that, that will have lots of milestones that deliver value for the organization, but will never stop that journey.
The other way to look at it is to say, long does it take to get started from that initial piece? Like giving you good understanding of all your people and how they're moving in and out of the organization and maybe one or two extra elements like costs or recruiting or something like that, that ties to, you know, a business imperative. The standard answer when you look at traditional analytics, how would you do this? You would build something called the data warehouse, maybe a data mart or, you know, put it in
data lake is the modern ways that people try to do this. There's pretty standard answers when you talk to analysts or customers or experienced system integrators in the analytics space, which is two years and $2 million. And so that can be pretty difficult for a large enterprise to be fair, but that can be a pretty difficult hurdle to get past. And it's part of why Visier even exists is to break that pattern.
by trying to pre-build a whole bunch of the work that otherwise people do one by one by themselves. The kind of difference between buying an apartment or building a house, one's much faster. And so, Visier, we generally advocate for somewhere in the three months area. You should be, and we can do it faster than that, depending on the scenarios. But to get you up and running with that core understanding of all your people and...
Dave Weisbeck (15:34.05)
the organization and move within the organization. So a couple of months.
Sean Luitjens (15:39.398)
And when they're going through that, are there a set of best practices when they're getting themselves ready and when they're kind of starting through to make sure that things go well? Because obviously they're taking a leap into people analytics, which is new. And so they can deliver value.
Dave Weisbeck (15:56.186)
Yeah, a couple of things to want everybody says their data is worse than everybody else's which I always find is a Statistically can't be true. So I always find that one a little bit of a Odd expression that everybody believes there's as worse than everybody else's and so their first practice I would advocate is everybody thinks you should clean up your data then do analytics You have to do it the other way
The only way you get clean data is through analytics. Because unless you bring visibility to the bad data, you'll never ever get good data. So there's no way to clean data in theory. You have to do it with the hard realities of exposing the bad data so people put in the energy to clean it up.
But it also becomes again one of these kind of lifestyle things and that people enter data and people will make mistakes and they answer bad data. They won't understand what they're supposed to put in this, you know, in this edit box when they interact with the system. So you have to have these like best practices of continuous hygiene of the data. So that's, that's definitely one of those practices. The second I would advocate is go after a specific, you valuable use case.
Ultimately to this idea, it's a journey. What you want to do is deliver something of value that the business goes, okay, this is awesome. We're better for this. And we will put in the energy. It can be funding and literal sense of budget, but more often than not, it's the investment of people's time and energy to get to the next phase. So to take on the next project.
and so pick something as well with a, there's a big change management as part of this. So pick something that is not blasting it to every single manager in an organization because that might be a difficult change management. So pick something that's slightly more narrow that you can be successful at. and then expand and expand and expand because often the technology is not the hardest part. It's actually the change management to bring, that kind of.
Dave Weisbeck (18:04.932)
philosophy of we will make decisions through data, bringing that to the company at large is actually the harder change.
Sean Luitjens (18:14.587)
Is there, I mean, I hadn't thought about that. guess two things. One, I hadn't even thought about the, it in before the data is clean. guess I, by default coming from comp, you spend the time to go clean the data before you drop it somewhere. So that's, that's actually very interesting because I would have not said it that way. As you go, the change management piece, is that more communication or is that more like getting people to not use anecdotal?
kind of black box gut feel make that change? is that where you see management being, have 30 years of experience and so I know better than what this number says?
Dave Weisbeck (18:54.17)
A lot of it is that, right? You get these beliefs. So you get beliefs that are best practices. Why do people leave organizations? Because they're underpaid, because they have bad managers, because the list goes on. But what does the data show? That, if you can just do the analysis that looks at the patterns of why people are leaving, that...
make a huge difference. But people find it, I don't know why, somehow comforting to go, I've been doing this for 20 years and I know that people tend to leave for this reason, so it must be true here. Or even, you know, to my point from before, I've tacked the basis of the claim. Is it even true that we have a problem with people leaving? Because people will often go with the, yes, but you know, Bob or Sally left and I really liked them and they left, therefore we have a problem.
But that was one person or two people. And the pattern shows that we're actually far better than the industry average when it comes to our ability to retain. And in fact, we're retaining our best people at a much higher level. That suddenly changes the conversation. But you find from an HR worldview, you need to have almost like that bravery around that. I think there's a reticence of like, you know,
Can I throw this back in the face of business people by bringing data? And so there's that confidence piece you gotta work towards, which comes from experience of using the data and having comfort in the data. And I think there's a cultural aspect of people going, that leader is in that position because they're smart and experienced. And so we should trust their judgment.
Yeah, but their judgment is informed by the wrong data, then we should help them by giving them the right data.
Sean Luitjens (20:52.968)
Okay, that's super interesting. Have you, kind of looping over to Total Rewards and so the core group, my anecdotal not data-backed experience over the past couple years is the, in our space, in particular in Total Rewards,
They don't either play well, work well, or aligned with, that they might know the people analytics team. And I think I was super surprised about that because comp is a highly analytical, you know, come up with the numbers, you know, all about the data and we're going to show you how smart we are with the data. I'll be at sometimes black boxish. and for some reason they're not aligned well. And I don't know what your thoughts are there because I'm surprised over the past while.
of how unlinked those two groups are.
Dave Weisbeck (21:44.89)
Yeah, I'm optimistic that I think it's going in the right direction. If anything, I would say that the total awards was further ahead. People Analytics has caught up and now I think the opportunity is to work more collaboratively together. So that would be my general view of it. But there are some really wonderful patterns that, from a total awards perspective,
As I said, they got to first and it's this idea of one of the most important ones is this idea of context to data Is the is somewhat maybe the fancy way to express it. What do I mean by that benchmark? And so the basic idea that that even if you apply it back again to say that turnover example is just on How do you know if it's good or bad? The reality is the only way to really understand if it's good or bad is to look at it relative
Is it worse than last year? Is it worse than our competitors or is it worse than the market or is it worse for this role in this industry, you know, in this location? Because all of that brings extra context if it's right. And Total Rewards Space got that very much right by going after, trying to understand and benchmark what are others paying for this role and this location and the other parameters we would look at.
But again, to that change management, I think there's an opportunity to also work together because ultimately your path to success there isn't for total rewards to say, this is right and lock it in a box. There's still a collaboration with the people leaders because they have other context and their context is more about the performance of this person or how important they are to the organization, how successful they are today and might make the organization in the future.
So there's always this kind of collaboration aspect. And here I think the people on the next teams and the total award teams can help each other because they both face a kind of a.
Dave Weisbeck (23:50.926)
And the last point would be, I think the other opportunity is more context to bring the data together. so compensation is, I always view it as somewhat of an economist. I took a few of those courses in school, but I can't say I'm an actual economist. But I bring that mindset to think about compensation as an incentive structure. And then, you now you want to bring that together with a larger view of the person.
that we might be able to get from the people analysis perspective and say, okay, how do we bring these two worlds together that can help each other? You get better insight into what you're trying to understand about how people are impacting the business when you bring that view in, but you can also make smarter decisions about gonna come right when you bring that larger context in so they can mutually help.
Sean Luitjens (24:42.066)
Yeah, think, I mean, I'll agree with all that. I think I'll just add, I think there's this...
It could be really good and I think it also freaks people out in comp, the ability to go back and check your work. So you have a hypothesis on what changes we make in pay or structure or benefits or LTIP programs and then we assume that's, they always assume they're right. And so the ability to kind of aim, fire, aim, fire, aim, fire is now here if you tie all the analytics together and or maximize our budget. But you have to have this kind of...
unwillingness or willingness to say i was wrong and in the past comp was a black box so i think there's been a lot of shift over them where people analytics is kind of comp was always like we're right and you don't get to see the data and if you're of my age i won't ask your age but you know if you're of my age you remember there's a point which you can even ask legally in the company what someone else made and hr would just tell you this is a number and you'll like it thing
And that's now changed to data is out there and it's there. And you have to admit like, actually, we didn't do this right. We thought we were going to pay here and people left or people didn't do this or didn't have the behavior. And now they have to do that. So I think there's huge opportunity with real-time analytics to make changes on the fly to comp programs to better align to business and pull in other data. But they also have to get over the fact that we had a theory. We were kind of right, but we were kind of wrong in these areas and adjust.
Dave Weisbeck (26:11.67)
Yeah, and that's a pretty normal cycle, right? So there's no, it's not really a negative, right, in terms of wrong. It's just more right now because you're constantly learning and evolving with these things. And even the programs that we think about in compensation have shifted and changed over time.
know, tech industry started with the stock options ideas and then moved to RSUs and like, and now there's a blend of multiple. you know, tech industry is reasonably sophisticated, finance industry, way more with these programs. And so, but the idea that you can measure them and understand are they actually creating the impact. I'm really fond of the idea of start with the end in mind.
work backwards to measure if you're actually achieving that goal.
Sean Luitjens (27:07.17)
so let me spin kind of one more direction since I know, selfishly, since I'm at Visier, we have the, the AI piece, but how do you see AI, albeit V for us, changing the people analytics business as you go forward. And so any analytics in general, but the analytics business with V or AI.
Dave Weisbeck (27:29.614)
Yeah, so I'll try to be succinct on this one. think I've not certain I've been.
Sean Luitjens (27:33.584)
No, I left you time. It'd be tough if I said you get 20 seconds. That would be a little rough.
Dave Weisbeck (27:38.97)
So first thing I would say, I go way back in time. started in college, my thesis that I wrote was on evolutionary approaches to artificial intelligence. And so my real lesson from that is there's this idea that you don't realize how hard something is unless you spend enough time on it to really understand it. And then you realize how hard it is. So I thought it was easy. Then I did the real work. And then I was like,
This is, it's going to be a long time until we ever get some real advancements in AI. So I started as a cynic. Second thing I would say is as it relates to analytics, even in the nineties, which now I am giving you a sense of how old I am. We had a dedicated research team trying to use natural language as an interface for analytics. And about every four years,
Somebody like went back and said, hey, wait a minute. What if we had natural language for analytics? And we've repeated this over and over and over again. And so that brings us to the present. First thing I'd say is I'm no longer a cynic. So what we have now in the large language models, I do believe will be very disruptive and not just in the ability to understand.
human give language and translate that into a technical what is the right information that I need, which was very fraught and hard to do, but also the ability for these some of these more recent large language models to really give you insight. Not to be just a fancy way to do search or to do lookup or I don't want to learn, know, SQL or SQL or whatever you prefer to pronounce that, but the, but really actually be able to
synthesize across data, find an insight from a lot of data. So I think it's going to be a dramatic change. Second part I'd relate, that was all the first part, the second part I'd relate is that I think it's also going to be massively disruptive to work. And at the end of the day, people analytics is really about understanding people in the frame of work and how do we make smarter decisions to get the work done, you know, more effectively, better, know, so we increase the outcomes of the business.
Dave Weisbeck (30:05.122)
And AI is going be really disruptive for work itself. And so we will find that just as robots have invaded the factory floor, so in that some sense, it's not AI, but it's form of automation. AI will also be a form of automation for the traditional office jobs. And so it'll start with augmenting, and then it'll start to do the work that some people do. And so we all have to get ready for that.
hopefully bright future, where we get a tremendous amount of productivity. You can definitely take a negative dystopian view, but I don't tend to follow that path. I tend to think that's how we progress as humanity. Really big picture. But we need to recognize it would be disruptive. So put them together now. Analytics and the way that we get information and insights fundamentally going to change. And it's really going to become the challenge we all have to take on is not how do we take a database of information.
make it sense to a human, how do we take a database of information and make it make sense to a large language model that can give proper insight now and relate to people and communicate with people in the way that they would expect to be communicated as if it was another person. And so it's going to change the way that we interface with information and at the same time it will disrupt work. And so now how do we get better insight to make smarter decisions about how we want to adapt.
And so that will really become what I think will be not just evolutionary, I think it'll be fairly revolutionary. And maybe our grandparents, since we're old, will have a radically different experience in the workforce than even our children.
Sean Luitjens (31:44.626)
Yeah.
Sean Luitjens (31:49.468)
Yeah, and I think, you the example I've been trying to use, because I agree with that, is the ability to do analytics so much faster. So if you go back way back to Lotus or you go back to Excel or to wherever, it was hard to do those analytics. so AI is going to change upon changes for HR. And so where you might've had entry-level customer support, you know, mid-level and manager, there might not be entry-level anymore.
Dave Weisbeck (32:09.156)
Yeah.
Sean Luitjens (32:13.896)
and so they're moving directly to the second one where they want to talk to a human because the AI is handling some of the support. You think about that in the comp space, well, what happened to that entry level job and the number of people and the people analytics that roll around that. And what we used to might take two or three years with surveys and survey submissions and data to come in to kind of get a flow. You'll be able to do that significantly faster. And I think the amount of data, like we talked about pulling in additional data, why I use customer support is number of calls.
Dave Weisbeck (32:30.842)
Yep.
Sean Luitjens (32:43.93)
of things that you do now can all be pulled in to look at people analytics and say, do I change with this thing that's changing everything and the humans that are involved with it? so I think having it natively in there to help you with the analytics that it would take us, like, I like to think both of us come from development. Like how much work some of these things would have been 20 years ago. you're like,
Dave Weisbeck (32:53.71)
Yep.
Sean Luitjens (33:06.556)
Like that would have been a quarter's worth of projects. And then just, how do I process that much data? If it's trillions of records of data to pull in, like, what do I run that on? And now it's like, well, I'll get it back to you. And if we're upset, if it's 500 milliseconds, like that's, can't believe it took us that long.
Dave Weisbeck (33:13.999)
Yeah.
Dave Weisbeck (33:23.448)
Yeah, and maybe to give a pragmatic, like, because I spoke a bit conceptually, but like, how will how we interface with information change? You know, way, way, way back when we created reports, which were just like, you know, lists of data. So like, we got them printed out in books, and we made sense of, okay, then we advanced, got a digital view, and we wanted to summarize, so we made dashboards.
but we didn't really know what to focus on. So then we created the KPI. So the idea that we would have a performance indicator. And so we'd traffic light them and we'd focus on the reds because everybody like if it's green, ignore it. If it's red, it's problem and go work on it. Now the world that we're not entering, we're in, it's already there. It's just, know, the grid was it, the romance or when I'm guessing like the future sphere is just not widely distributed. So what's very easy to do today is just not widely deployed.
is just ask the AI, should, for whatever the business concern is that that dashboard relate to or KPIs, just ask it. What should I focus on? What are the issues that I should spend time on?
Sean Luitjens (34:34.348)
What are the levers that move this number, which, you know, again, used to would have taken a lot of modeling, putting in different places and making changes. It's a, it's that quick now. And I think it's, I fundamentally think in people analytics and in total wars in particular, it's kind of truly allow them to be, come up with more strategy. In fact, my answer has been, I apologize for those of us in dev that are kind of creating this world for you because what used to be your strategy.
What you can build is a strategy that's limited by technology, so you don't have to think that hard. You actually have to do a lot of thinking now creatively and strategically to come up with a cool strategy, because we're going to give you lot of analytics around what changes the business.
Dave Weisbeck (35:12.196)
Yep. Yeah. And in the total reward space, you would ask the, once the systems are plugged in, right, as I said, it's not widely distributed just as yet, the question maybe we want to pay for performance culture. Where, what are the issues that are stopping us from achieving that? It's not the dashboard, it's not the report, it's not the sea of data that you're coming through. You ask the system to tell you the answer.
So it's a little bit Star Trek, you know, computer.
Sean Luitjens (35:45.032)
That's better, I come up with SkyNet but that doesn't go so well so I... Yeah.
Dave Weisbeck (35:47.482)
Yeah, it's kind of, it's a little dystopian, but, you know, but yeah, a little Star Trek and, you know, we just hit the little communicator button and off we go.
Sean Luitjens (35:57.746)
This is awesome. The one question asked doesn't have to be people analytics at the end is if you could automagically solve anything in all of HR, what would it be?
Dave Weisbeck (36:08.474)
Automatic change. So I have this worldview that finance went first. And what I mean by that, finance used to be the accounting department. And then they added in the whole layer of what it's generally referred to as FP &A. And so they became very important for the overall like...
planning of the business. So I have a worldview that like, I feel almost like we've been on the cusp of that in an HR world, but we still just for whatever reason, we just can't get over the, you know, that last little bit of hurdle. We just need the straw apparently for the camel's back. the, and so if I could change one thing, it would be to like cement into every organization that they have whatever we might call it.
because there's a tremendous amount of work that HR has to do to like the accounting department by analogy, to make certain that the business continues to function and operate. But people are the single greatest impact to the success of a business. They sell everything, they create everything, they deliver everything, they, you know, everything that they operate and service. people are the key to every business, the single most important thing.
But I think the strategy is around people that's still been lightly served. And so if I could have my one wish, it'd be to really cement that in place. I don't know what to call it. We couldn't call it the FPNA, obviously, but like the, I don't know what to call it. I won't make up a name. that kind of that, where all of the strategy and intelligence and data, that that has an equal footing to the operational side of HR.
Sean Luitjens (38:03.246)
Okay, that's good. It's similar. used say, know, HR for years, to your point, has been saying they want a seat at the table. And I've been saying, you know...
they've had a seat, it's just been a folding chair. What they really want is the strategic chair at the table to help make decisions. And so I think that's it. So this has been great, great job. You're the first Visier person. I think it's super helpful. I know we get tons of questions around people analytics. So I think this gives us a great start and really appreciate you taking time out.
Dave Weisbeck (38:23.087)
Yeah.
Sean Luitjens (38:36.904)
your schedule too. So although it was easier to book with you than others, I could see, you know, couldn't, it was harder for you to hide.
Dave Weisbeck (38:41.434)
Yeah, exactly. Well, hopefully helpful to everyone, hopefully enjoyable and, you know, listen in for people. And yeah, hopefully get some good feedback and happy to come back if it would be viable to do so in future.
Sean Luitjens (38:46.33)
Yeah, so for everyone else.
Sean Luitjens (39:00.712)
Awesome. And so for everyone else, follow on Work Defined, follow on our site, YouTube site, or the blog, and we will look for you next time.
